Kafka, Samza, and the Unix Philosophy of Distributed Data
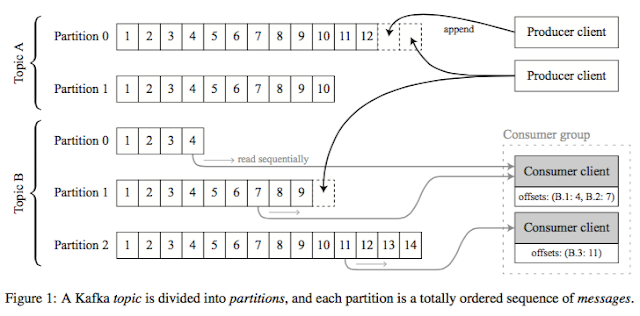
This paper is very related to the "Realtime Data Processing at Facebook" paper I reviewed in my previous post. As I mentioned there Kafka does basically the same thing as Facebook's Scribe, and Samza is a stream processing system on Kafka. This paper is very easy to read. It is delightful in its simplicity. It summarizes the design of Apache Kafka and Apache Samza and compares their design principles to the design philosophy of Unix, in particular, Unix pipes. Who says plumbing can't be sexy? (Seriously, don't Google this.) So without further ado, I present to you Mike Rowe of distributed systems. Motivation/Applications I had talked about the motivation and applications of stream processing in the Facebook post. The application domain is basically building web services that adapt to your behaviour and personalize on the fly, including Facebook, Quora, Linkedin, Twitter, Youtube, Amazon, etc. These webservices take in your most recent actions (likes, cli