Paper Summary: DeepXplore, Automated Whitebox Testing of Deep Learning Systems
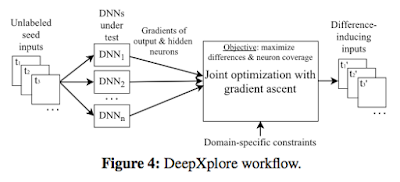
This paper was put on arxiv on May 2017, and is authored by Kexin Pei, Yinzhi Cao, Junfeng Yang, Suman Jana at Columbia and Lehigh Universities. The paper proposes a framework to automatically generate inputs that trigger/cover different parts of a Deep Neural Network (DNN) for inference and identify incorrect behaviors. It is easy to see the motivation for high-coverage testing of DNNs. We use DNN inference for safety-critical tasks such as self-driving cars; A DNN gives us results, but we don't know how it works, and how much it works. DNN inference is opaque and we don't have any guarantee that it will not mess up spectacularly in a slightly different input then the ones it succeeded. There are too many corner cases to consider for input based testing, and rote testing will not be able to cover all bases. DeepXplore goes about DNN inference testing in an intelligent manner. It shows that finding inputs triggering differential behaviors while achieving high neuron cover