Clock-SI: Snapshot Isolation for Partitioned Data Stores Using Loosely Synchronized Clocks
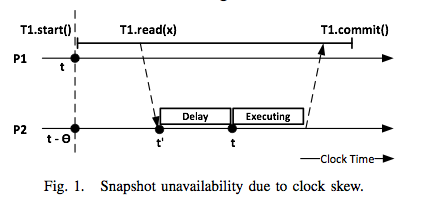
This paper appeared in SRDS 2013, and is concerned with the snapshot isolation problem for distributed databases/data stores. What is snapshot isolation (SI)? (I took these definitions almost verbatim from the paper.) SI is a multiversion concurrency control scheme with 3 properties: 1) Each transaction reads from a consistent snapshot, taken at the start of the transaction and identified by a snapshot timestamp. A snapshot is consistent if it includes all writes of transactions committed before the snapshot timestamp, and if it does not include any writes of aborted transactions or transactions committed after the snapshot timestamp. 2) Update transactions commit in a total order. Every commit produces a new database snapshot, identified by the commit timestamp. 3) An update transaction aborts if it introduces a write-write conflict with a concurrent committed transaction. Transaction T1 is concurrent with committed update transaction T2, if T1 took its snapshot before T2 comm