Megastore: Providing scalable, highly available storage for interactive services
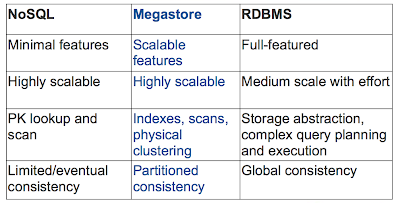
Google's Megastore is the structured data store supporting the Google Application Engine . Megastore handles more than 3 billion write and 20 billion read transactions daily and stores a petabyte of primary data across many global datacenters. Megastore tries to provide the convenience of using traditional RDBMS with the scalability of NOSQL: It is a scalable transactional indexed record manager (built on top of BigTable), providing full ACID semantics within partitions but lower consistency guarantees across partitions (aka, entity groups in Figure 1). To achieve these strict consistency requirements, Megastore employs a Paxos-based algorithm for synchronous replication across geographically distributed datacenters. I have some problems with Megastore, but I save them to the end of the review to explain Megastore first. Paxos Megastore uses Paxos , a proven, optimal, fault-tolerant consensus algorithm with no requirement for a distinguished master. (Paxos is hard to cover i...